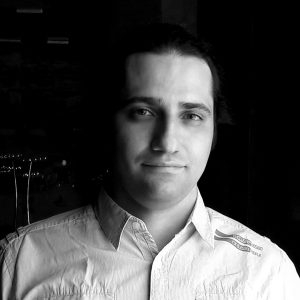
Meysam’s PhD project aims to develop a machine learning (ML) model to evaluate building performance in the early architectural design stage, considering architectural design features and smart services. Buildings equipped with smart services and smart control strategies can reduce energy consumption and increase energy flexibility. Among various smart services and control strategies, certain ones, such as shading device control, window opening control, and indoor temperature setpoint control, directly interact with a building’s architectural features, such as window size, window SHGC, and building thermal mass. The specifications of building components and the control strategies of smart services can mutually influence each other’s performance, consequently impacting the overall performance of the building. Therefore, to achieve high building performance, it is important to consider these smart services and building design together during the design process.
During the early architectural design stage, multiple simulations, sensitivity analyses, and optimizations are typically conducted to make decisions regarding various building components. The addition of variables related to smart services at this stage can greatly increase the number of required simulations, the complexity of the modelling, the simulation time, and the need for computer resources. Employing data-driven methods such as machine learning (ML) can offer a viable alternative to intricate simulations during this design stage.